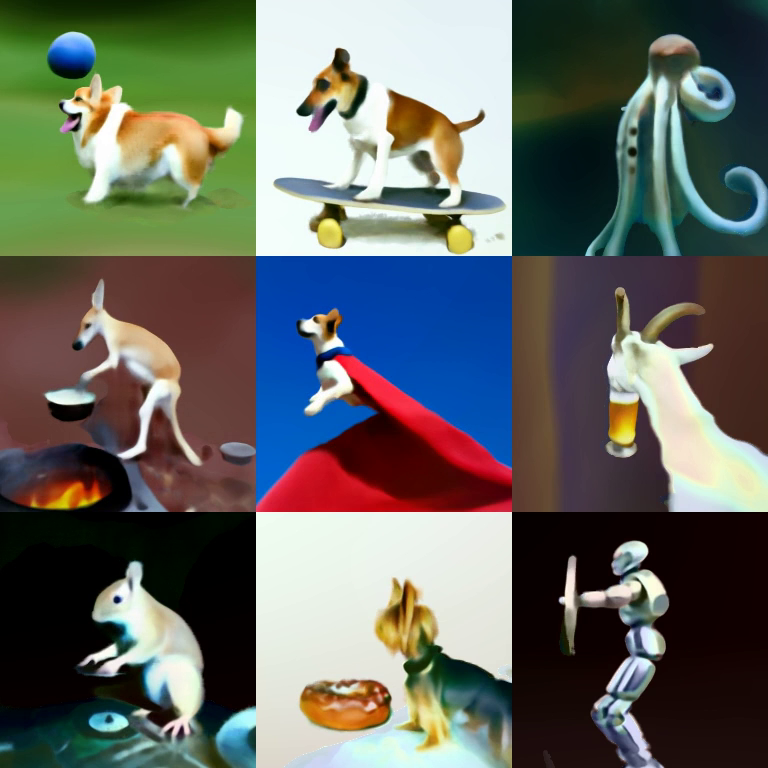
Text-To-4D Dynamic Scene Generation
🌟 Introducing Text-To-4D Dynamic Scene Generation! 🤖✨ - Creates 3D dynamic scenes from text descriptions - Utilizes a 4D dynamic Neural Radiance Field (NeRF) - Optimizes scene appearance, density, and motion consistency - Offers dynamic video outputs viewable from any angle - Requires no 3D or 4D data input - Leading the way in generating 3D scenes from text descriptions 🚀🎥 #AI #DynamicScenes #TextTo4D
- The MAV3D method generates 3D dynamic scenes from text descriptions using a 4D dynamic Neural Radiance Field (NeRF).
- Scene appearance, density, and motion consistency are optimized by querying a Text-to-Video (T2V) diffusion-based model.
- The dynamic video output from text can be viewed from any camera location and composited into any 3D environment.
- MAV3D does not require 3D or 4D data; the T2V model is trained on Text-Image pairs and unlabeled videos.
- The method showcases improvement over established internal baselines in quantitative and qualitative experiments.
- It is the first method known to generate 3D dynamic scenes from text descriptions.
- Various scenarios like a corgi playing with a ball or a space shuttle launching can be generated using this approach.
- The method involves loading meshes corresponding to text descriptions like a panda dancing or a clown fish swimming.
- The Image-to-4D process allows for input images to be transformed into dynamic scene videos.
- The citation for the work is available in the provided format.